Select a topic
Rethinking How People Move in Cities November 14, 2014
Using Crowd Modelling and Real-Time Analytics to Manage Our Increasingly Crowded Cities
Mobility models are typically one of either of these two types: ‘typical day’ models, e.g. using spatial population and employment data to model how individuals spread out across a transport network to utilise available capacity during their travel-to-work journeys, or mass exodus resulting in extreme crowding and congestion in a disaster scenario. Neither of these modelling regimes describe our everyday urban mobility challenges especially well.
Dr. Anders Johansson, CTO and co-founder of Crowd Vision, looks at how observations and analysis of empirical crowd behaviour can be used to formulate a theory of crowd behaviour, which will identify important links from the micro to the macro scale. This will then form the basis of predictive modelling tools that when combined with real-time sensing can be used to regulate and optimise the movement of pedestrians in crowded environments.
Right, so I’m not an architecture, I’m an engineer by training and I will talk about technology mostly and mostly about crowd modelling and real time sensing of crowds. One thing I do have in common with most of you, I think it’s the fascination about spaces and how people use space on different scales.
This is something that came up in several presentations in the morning, which is the notion of densification and the basic density on different scales. And this is the population density of England. And you can see it quite clear, cluster within the red one, which is obviously London. So people don’t really spread out in space, they tend to cluster quite extremely in different areas and if you then zoom in to this area which is London and look at the city scale, one scale down, and look at the usage of the roads, in the Greater London Authority area and color code them, you can see that a small faction of roads are used extremely much and the vast majority is not used as much. It’s a similar sort of extreme distribution. If you then zoom in even further in parts of the city, you see quite similar kind of patterns emerging where parts of the space are really crowded and some, you know, gaps in other places. So I am quite fascinated looking at different scales, to see how they link to each other, and of course, you can also measure, because as we heard in previous talks, there’s lots of data now, lots of data coming from various sources, various scales and you can basically measure the usage of space in different scales and you can plot the distributions and see quite similar patterns emerging on the various scales, see also the hot spots in time and space, that are extremely crowded, other points in space and other times, it’s not that crowded.But this of course are just snapshots of the evolutionary process. There is some sort of mechanism that leads up to those snapshots, and of course then, an interesting question is “What sort of social mechanisms that lead to these sort of outcomes at a higher scale?”
And depending on which scale you look at you get different sort of social mechanisms, or human mechanisms that are responsible for these outcomes, starting with the local scale, these kind of crowd patterns really come out of routine decisions, so second by second, minute by minute decisions to kind of navigate spaces, so it’s a really routine thing. And as it turns out, people are quite efficient at the local scale and one scale up which is the, kind of city scale. The main human decision behind this is the route choice so basically which route you take from A to B. And at the highest, sort of, population density scale, then is really migration, urbanisation, which is basically once in a lifetime, very kind of rear event to move from one area to the others. There are different temporal scales and different spatial scales.
Right. So the question is then, what are the tools being available to us, trying to make sense of all of this? And when we started this research, more than 10 years ago, the kind of standard tool used by architects, by planners and so on is something called the fundamental diagram, which is this dashed nice curve here, which maps the density of the crowds, so basically the numbers of persons per square metre to the correspondent flow rate, which is how many persons flow across a line of a metre width per second. It’s a very neat sort of view of the world, so it’s a neat line there. And obviously, once you start to measure, the reality is much more messy. So depending on where you measure, so where in the world you measure, geographically and in what context, you get quite different looking curves coming out of it. For example, the red mark is straight from our own measurements in Saudi Arabia, the green ones are from a study in Germany, the blue ones are from Japan, so depending on where you measure, you get bit different looking curves that make it quite hard to use this in a planning process because basically you need to first design and build and the use the space and then you measure to what looks like, and that is already too late, you know. So, from the design process, you obviously, if you measure different places and get quite different results, it’s not a very good tool for predicting outcomes.
So that’s one of the problems. The other problem is low and medium, sort of, density levels have been really well researched by many people around the world so it’s quite a lot of good data for this level. But very high crowd density, as it turns out, for obvious reasons, hasn’t been many measurements there. So what people did is basically extrapolate this line and lets assume it almost goes down to zero. Because if it’s crowded enough, you can’t quite move around any more, because you have to slow down and every single [inaudible] stop. That was the theory of back then. Having measured really dense crowds, it turns out its not a case actually, everything is not just in a freezing to standstill for very dense crowds. So this is from a study we did in Saudi Arabia during the pilgrim to Hajj, the Hajj pilgrimage to Mecca, which is one of the most crowded places on the planet. So what happens is, as the crowd does this building up, the flow is dropping, but then at some point, people are starting to move again, but not in a kind of regular smooth fashion rather, you can see these waves of propagating through the crowds, basically a transition point from a nice flowing crowd to a stop-and-go waves propagating through the crowds. It’s actually very dangerous because these waves are packing the crowds even denser and denser and becomes very dangerous, and of course you can also measure it. So we can see here we measure the density of the crowd as a function of time and space. And you can see these red lines, which are the density waves, you know, going through the crowds here. And sad enough this was not the end of the story. So as the crowd density was building up more and more, we actually found a second transition from stop-and-go waves to something called “crowd turbulence”. So you can see here, people are being moved around by the crowd in all sort of different directions so this is actually very, very dangerous because this is just 10 minutes before lots of people died, there’s hundreds of people actually died in this place from the sheer crowd density, it’s a very dangerous sort of patterns there.
And similar to the stop-and-go waves, you can of course measure this by technology and you can measure the spatial-temporal dynamics of the crowds in these settings. Going back to the question we asked before which is “Given these interesting patterns, some of them very dangerous, but these patterns nevertheless on a macro level, what are the sort of, underlying local scales, micro-mechanism leading up to the macroscopic patterns?” And to be able to answer this question, of course you need empirical data on how people move around on, a kind of, fine scale. So we developed software to track individuals as they move around in different spaces to get different [inaudible] of them. Then we could use this data to basically extract the underlying interaction mechanisms between [inaudible] or pedestrians. An also interesting mechanism that occur, emerge from this work. One is, if you look at the strengths, the magnitude of interaction, as a function of the angle of interaction. As it turns out, people react to what happens in a kind half circle in front of them, and what happens behind their the backs not so much bothered by. So basically people react to what happens in this, kind of, half circle in front of them. And if we measure the interaction magnitude as a function of the distance between persons is this nice exponential decay so people react much more strongly to what happens close to them. Because we want to keep our private space around ourselves and so on.
And another thing that emerge from the local measurements is that, one thing that came out across different data set which seems to be some sort of unifying mechanism is the time headways, so basically follow someone, it seems that the time headway keeps the person in front of you is about half of second, and we saw this value again and again in different studies, it seems to be something, even though some measurements differ between cultures and context and geographies. It seems this time headway, seems to be quite static around the different measurements and indeed, rather measuring of flow rates, you measure the time headways. See all these curves here, basically collapsed on to the same line which is the half second line. So at this point, we realised what one of the sort of unifying underlying local mechanisms are, then this creates a link from the micro scale, up to the macro scale and then you can generalise your system and create one sort fit curve that fits all of the different data sets. Then you get more of the kind of a predictive power coming out of this. You can actually use it as a predictive tool, you don’t really need to measure every single situation before you try to influence it.
So this was the link from the micro to the macro scale, there is also turns out a link back from the macro to the micro again, and to illustrate this, this is a situation where we have a bidirectional flow in a shopping mall, and if you look carefully in this, actually, it’s just not a random mix of people, if you look carefully, you see they separate in two or three different lanes of uniform direction. And if you want to stop a person and ask “Why did you decide to organise yourself in this sort of pattern?”, they wouldn’t be aware of that they are actually forming these patterns. So the one is planning it is just emerges out of the local scale interactions and some of these patterns can be sustained for a quite long time. And to illustrate this, you can have trails being formed in grass and snow, and so on. Because, obviously, if we want to pass from one side to the other, it’s easy to follow in the footsteps of someone else and to basically initially, you have some randomness and then, once you get some path, it tends to be reinforced over time. And again, as it turns out, some of these things, can be reinforced over many, many years.
And if you look at the evolution of road networks, this is just one example of the city of Milan, but if you look in almost any other city and look at kind of snapshots of the road network over time, you can realise that some of the main arteries in modern cities used to be almost like the foot paths a few hundreds years ago, so you can say this, first you get these reinforced part and then, you know, it gets reinforced by time and then, someone puts asphalt on the ground and that’s how kind of becomes a multilane motorway. So you can see how these things evolve over hundreds of years, it’s quite a strong mechanism in that sense.
So this was my first part of my talk, which is being academic I feel I need to give you some theory and some background, I also recognise that it’s nice to talk about applications, the usefulness of these kind of theories and models. So we’ll briefly talk about a few different applications of this work. The first one which may be an obvious one for most of you is of course once you identify some of these microscopic, you know, social mechanisms, you can put them together into models, you can then write a piece of software and then you can, you know, speculate and model, what cityscapes how they might function, you can model urban areas, mobility in urban areas, and mobility in buildings and so on. So basically, putting these equations, these models together, in a piece of software. And of course, modelling is more a predictive tool, something you can predict how an architecture, any other sort of change might influence the way people move around in spaces, but it can also be real time tools. If you measure, if you use a crowd sensor to measure how humans move around in real time, and fuse it together with models, you can actually combine the two; and this is one example of a real evacuation of Canary Wharf, that was mentioned before. Where we had a few point detectors, so basically a few video cameras in different places in space, so we had a very good detailed information of how many persons where in different locations, and how they moved around. And then we could fill the space between those sensors, using modelling tools. So you can see here that people are flowing out from the buildings during an evacuation drill and getting to the master point and you can see the tube station there and now people are moving back into the buildings again. So you can see these waves of people going in and out of the buildings and then coming back again. So this is a really merging, or fusing together, predictive modelling with real time sensing.
And going back to the example I mentioned before, which was the pilgrimage to Mecca, this something we have studied for last ten years or so and before that was a very sad history of large crowd disasters. Every couple of years there were hundreds of people dying from the sheer amount of crowds and since we got involved with implement lots of different changes, of course there are structural changes, there’s been a lot of architectural, planning changes, but also quite a lot of management changes. And there is also now quite a lot real time measurements, of course, it’s one thing to analyse crowds, to record a crowd, analyse it to come up with some insight afterwards, but if you measure these very dangerous places, it’s of course, better if you can measure real time so that your insights can fit into decision making process, as you can actually change the course of the events rather than coming up with the results, you know, a few months or years later. And this is exactly what has been done, so now, around 300 CCTV cameras being linked to a piece of software, that measures how people move around the space. So it’s basically, the planned event how people are supposed to move around different places and there’s actually measurements and if they’re looking different means you may want to interfere and try to proactively manage the crowd, redirect pieces, or parts of the crowd or interfere in one way or the other, to manage this situation rather than waiting for the situation to be really bad and then it may be too late to interact.
Another example is a more recent example, which is a project we did at London City Airport, where we measured basically, you know, square metre by square metre, second by second, how people move around in spaces. So we measured journey times, queue times, density hotspots to try to manage the crowds in real time, to try to prevent, to try to speed up the flow through the airport. So the previous example was more kind of spot measurements with gaps in between this is a more like fill a large amount of space with sensors which can measure what’s going on across a larger space. And my final slide is linking this mobility modelling into other areas because obviously a various different areas, it’s a link to mobility one way or the other. And if you look at congestion, it’s a very localised problem, you can draw a nice tight boundaries around the problem areas, and you can study it. If you look at some other problem areas, such as the spread of disease, you can’t quite draw a boundary around the spread of disease, because in a global city like London of course, there are connections to the rest of the world so you can’t just study the spread of disease in a localised area, in London, and we’ve been putting together some hypothetical models of how this might work which is basically to link the global flights system, system of flights, going across the airports into London and then see how, various diseases might spread through this network and then once inside London, you can model it locally how this disease spread around. So the heat map there would be the hot spot of diseases, and it seems one of the hot spots are actually quite close to this place, but again, it’s just a hypothetical situation, it’s nothing like ebola, anything like this is just, you know, computer games.
Ok, thank you very much!
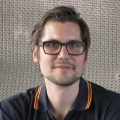
Dr. Anders Johansson
Senior Lecturer in Systems Engineering at the University of Bristol, CTO of Crowd Vision, Honorary Senior Research Associate of UCL’s CASA, and a member of the WHO Virtual Interdisciplinary Advisory Group on Mass Gatherings; Dr Johansson researches and develops mathematical models and real-time sensing and analytics tools to study the interaction of crowds, infrastructure and technology.
Comments